AI Training: A Beginner's Guide to Getting Started
Understanding the Basics of AI Training
Artificial Intelligence (AI) has become an integral part of various industries, offering innovative solutions and improving efficiencies. However, the concept of AI can be daunting for beginners. This guide aims to simplify the process of getting started with AI training by breaking down the fundamental aspects you need to know.
At its core, AI training involves teaching machines to mimic human intelligence by learning from data. The process includes data collection, data preprocessing, model selection, training, and evaluation. Understanding these steps is crucial for anyone looking to dive into AI.

Essential Components of AI Training
Data Collection and Preprocessing
The first step in AI training is data collection. Data serves as the foundation for AI systems, and the quality and quantity of this data greatly impact the performance of the AI model. Once collected, data often needs to be cleaned and formatted, a process known as preprocessing. This step ensures that the data is consistent and ready for analysis.
Model Selection
Choosing the right model is another critical component of AI training. Various models are available, each with its own strengths and weaknesses. Some common models include decision trees, neural networks, and support vector machines. The choice of model depends on the specific problem you are trying to solve and the nature of your data.

Training and Evaluation
The Training Process
Once a model is selected, it’s time to train it using the prepared data. During this phase, the model learns to recognize patterns and make predictions or decisions based on the input data. This step may require significant computational resources, depending on the complexity of the model and the size of the dataset.
Model Evaluation
After training, it is essential to evaluate the model's performance. This involves testing the model with a separate set of data that was not used during training. Evaluation metrics such as accuracy, precision, recall, and F1 score help determine how well the model is performing and whether any adjustments are necessary.

Challenges and Considerations
AI training is not without its challenges. One common issue is overfitting, where a model performs well on training data but poorly on unseen data. This can be mitigated through techniques like cross-validation and regularization. Additionally, ethical considerations such as data privacy and bias must be addressed to ensure responsible AI development.
Understanding these challenges is key to developing robust and ethical AI systems. As you gain experience, you will learn to navigate these obstacles more effectively.
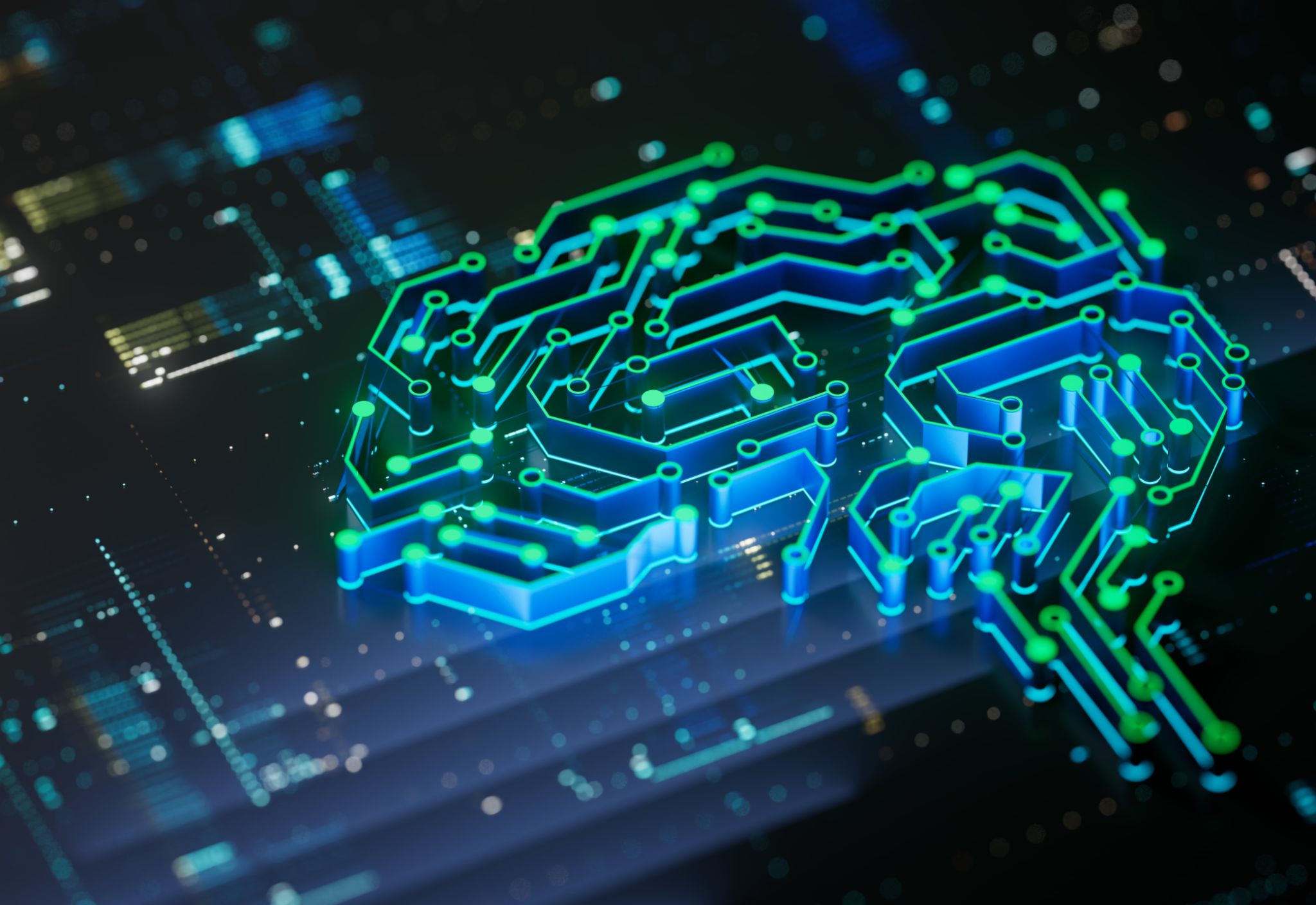
Getting Started with AI Training
For beginners looking to start their journey into AI, various resources are available. Online courses, tutorials, and workshops can provide valuable knowledge and hands-on experience. Platforms like TensorFlow and PyTorch offer tools and libraries that simplify the process of building and training AI models.
With dedication and practice, you can develop the skills needed to harness the power of AI in your field of interest. As technology continues to evolve, staying informed about the latest advancements will help you remain competitive in this dynamic industry.